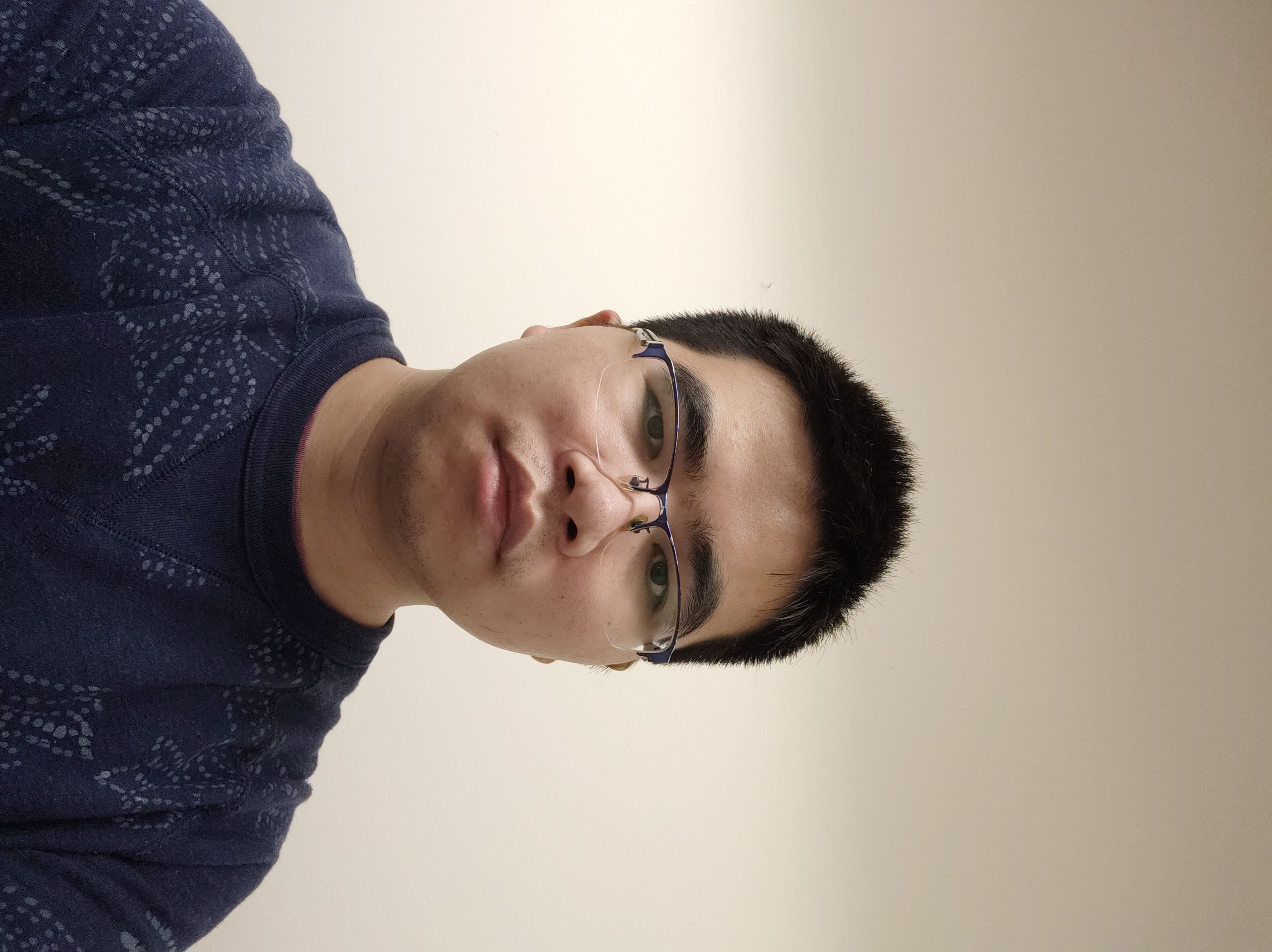
Changlong Wu
Visiting Assistant Professor
Department of Computer Science
Purdue University
Email: changlong1993 at gmail.com
Google Scholar: View Profile
I'm a Visiting Assistant Professor at the NSF Center for Science of Information (CSoI) and Department of Computer Science at Purdue University, working closely with Prof. Wojciech Szpankowski and Prof. Ananth Grama. I earned my Ph.D. degree in Electrical Engineering from the University of Hawaii at Manoa, where I was advised by Prof. Narayana Prasad Santhanam. Before that, I earned my Bachelor's degree in Computer Science from Wuhan University.
I will be joining the Department of ECE at the University of Arizona as an Assistant Professor starting Fall 2025.
Research Interests
My research interests span a wide range of algorithmic and theoretical aspects within machine learning, statistics, and information theory. Specifically, I am focused on exploring the learnability (both qualitatively and quantitatively) across various machine learning paradigms. At present, my work delves into areas such as online learning, estimation theory, and the foundational elements of trustworthy machine learning.
Selected Publications
- C. Wu, A. Grama and W. Szpankowski. No Free Lunch: Fundamental Limits of Learning Non-Hallucinating Generative Models. In International Conference on Learning Representations (ICLR). 2025.
- C. Wu, A. Grama and W. Szpankowski. Information-theoretic Limits of Online Classification with Noisy Labels. In Conference on Neural Information Processing Systems (NeurIPS). 2024.
Extended Journal version available from arXiv.
- C. Wu, J. Sima and W. Szpankowski. Oracle-Efficient Hybrid Online Learning with Unknown Distribution. In Conference on Learning Theory (COLT). 2024.
- C. Wu, Y. Wang and A. Grama. A Theory of Fault-Tolerant Learning. In International Conference on Machine Learning (ICML). 2024. (Spotlight, acceptance 3.5%).
- C. Wu, A. Grama and W. Szpankowski. Online Learning in Dynamically Changing Environments. In Conference on Learning Theory (COLT). 2023.
- C. Wu, M. Heidari, A. Grama and W. Szpankowski. Expected Worst Case Regret via Stochastic Sequential Covering. In Transactions on Machine Learning Research (TMLR). 2023.
- C. Wu, Y. Wang, A. Grama and W. Szpankowski. Learning Functional Distributions with Private Labels. In International Conference on Machine Learning (ICML). 2023.
- C. Wu, M. Heidari, A. Grama and W. Szpankowski. Precise Regret Bounds for Log-loss via a Truncated Bayesian Algorithm. In Conference on Neural Information Processing Systems (NeurIPS). 2022. (Oral, acceptance 1.8%)
Extended Journal version in IEEE Transactions on Information Theory (TIT). 2023.
- C. Wu and N. Santhanam. Prediction with Finitely many Errors Almost Surely. In International Conference on Artificial Intelligence and Statistics (AISTATS). 2021.
Extended Journal version available from arXiv.
- C. Wu and N. Santhanam. Non-uniform Consistency of Online Learning with Random Sampling. In International Conference on Algorithmic Learning Theory (ALT). 2021.
Professional Services
Journal Reviewer: IEEE Transactions on Information Theory 2018 - present; Transactions on Machine Learning Research 2024 - present; Journal of Machine Learning Research 2025.
Conference Reviewer: ISIT 2018-2021, 2023-2024; AISTATS 2021; TheWebConf 2023; NeurIPS 2023-2025; ALT 2024-2025; ICLR 2024-2025; ICML 2024-2025; COLT 2025.
All Publications
Note: * indicates equal contribution, and (α-β) indicates alphabetical order.Preprints
- P. Devulapalli*, C. Wu*, A. Grama, and W. Szpankowski. Agnostic Continuous-Time Online Learning. Submitted. 2025.
- C. Wu, A. Grama and W. Szpankowski. Robust Online Classification: From Estimation to Denoising. Submitted. 2024.
- M. Drmota, P. Jacquet, C. Wu, and W. Szpankowski. Precise Regularized Minimax Regret with Unbounded Weights. Submitted. 2024.
- C. Wu, and N. Santhanam. Prediction with Eventual Almost Sure Guarantees. Submitted. 2024.
Journal Papers
- C. Wu, M. Heidari, A. Grama and W. Szpankowski. Regret Bounds for Log-loss via Bayesian Algorithms. In IEEE Transactions on Information Theory (TIT). 2023.
- C. Wu, M. Heidari, A. Grama and W. Szpankowski. Expected Worst Case Regret via Stochastic Sequential Covering. In Transactions on Machine Learning Research (TMLR). 2023.
Conference Papers
- (α-β) N. Papagiannis, W. Szpankowski and C. Wu. Online Learning with Nasty Experts. In IEEE International Symposium on Information Theory (ISIT). 2025. To appear.
- C. Wu, A. Grama and W. Szpankowski. No Free Lunch: Fundamental Limits of Learning Non-Hallucinating Generative Models. In International Conference on Learning Representations (ICLR). 2025.
- C. Wu, A. Grama and W. Szpankowski. Information-theoretic Limits of Online Classification with Noisy Labels. In Conference on Neural Information Processing Systems (NeurIPS). 2024.
- C. Wu, J. Sima and W. Szpankowski. Oracle-Efficient Hybrid Online Learning with Unknown Distribution. In Conference on Learning Theory (COLT). 2024.
- C. Wu, Y. Wang and A. Grama. A Theory of Fault-Tolerant Learning. In International Conference on Machine Learning (ICML). 2024. (Spotlight, acceptance 3.5%).
- M. Drmota, P. Jacquet, C. Wu, and W. Szpankowski. Minimax Regret with Unbounded Weights. In IEEE International Symposium on Information Theory (ISIT). 2024.
- J. Sima*, C. Wu*, O. Milenkovic, and W. Szpankowski. Online distribution learning with local privacy constraints. In International Conference on Artificial Intelligence and Statistics (AISTATS). 2024.
- C. Wu, A. Grama and W. Szpankowski. Online Learning in Dynamically Changing Environments. In Conference on Learning Theory (COLT). 2023.
- C. Wu, Y. Wang, A. Grama and W. Szpankowski. Learning Functional Distributions with Private Labels. In International Conference on Machine Learning (ICML). 2023.
- C. Wu, M. Heidari, A. Grama and W. Szpankowski. Precise Regret Bounds for Log-loss via a Truncated Bayesian Algorithm. In Conference on Neural Information Processing Systems (NeurIPS). 2022. (Oral, acceptance 1.8%)
- C. Wu, M. Heidari, A. Grama and W. Szpankowski. Sequential vs. fixed design regrets in online learning. In IEEE International Symposium on Information Theory (ISIT). 2022.
- C. Wu and N. Santhanam. Estimating Properties of Dynamic Graphical Models. In IEEE International Symposium on Information Theory (ISIT). 2021.
- C. Wu and N. Santhanam. Non-uniform consistency of online learning with random sampling. In International Conference on Algorithmic Learning Theory (ALT). 2021.
- C. Wu and N. Santhanam. Prediction with Finitely many Errors Almost Surely. In International Conference on Artificial Intelligence and Statistics (AISTATS). 2021.
- C. Wu and N. Santhanam. Entropy property testing with finitely many errors. In IEEE International Symposium on Information Theory (ISIT). 2020.
- C. Wu and N. Santhanam. Almost uniform sampling from neural networks. In Annual Conference on Information Sciences and Systems (CISS). 2020.
- C. Wu and N. Santhanam. Being correct eventually almost surely. In IEEE International Symposium on Information Theory (ISIT). 2019.
- C. Wu, W. Chen, and J. Zhang. Greedy algorithm with approximation ratio for sampling noisy graph signals. In IEEE International Conference on Acoustics, Speech and Signal Processing (ICASSP). 2018.
- C. Wu, M. Hosseini, and N. Santhanam. Redundancy of unbounded memory markov classes with continuity conditions. In IEEE International Symposium on Information Theory (ISIT). 2018.
- K. Oshiro*, C. Wu* and N. Santhanam. Jackknife estimation for markov processes with no mixing constraints. In IEEE International Symposium on Information Theory (ISIT). 2017.
Dissertation
- Online Learning and Prediction with Eventual Almost Sure Guarantees. University of Hawaii at Manoa. 2021.